Is LLM progress slowing?
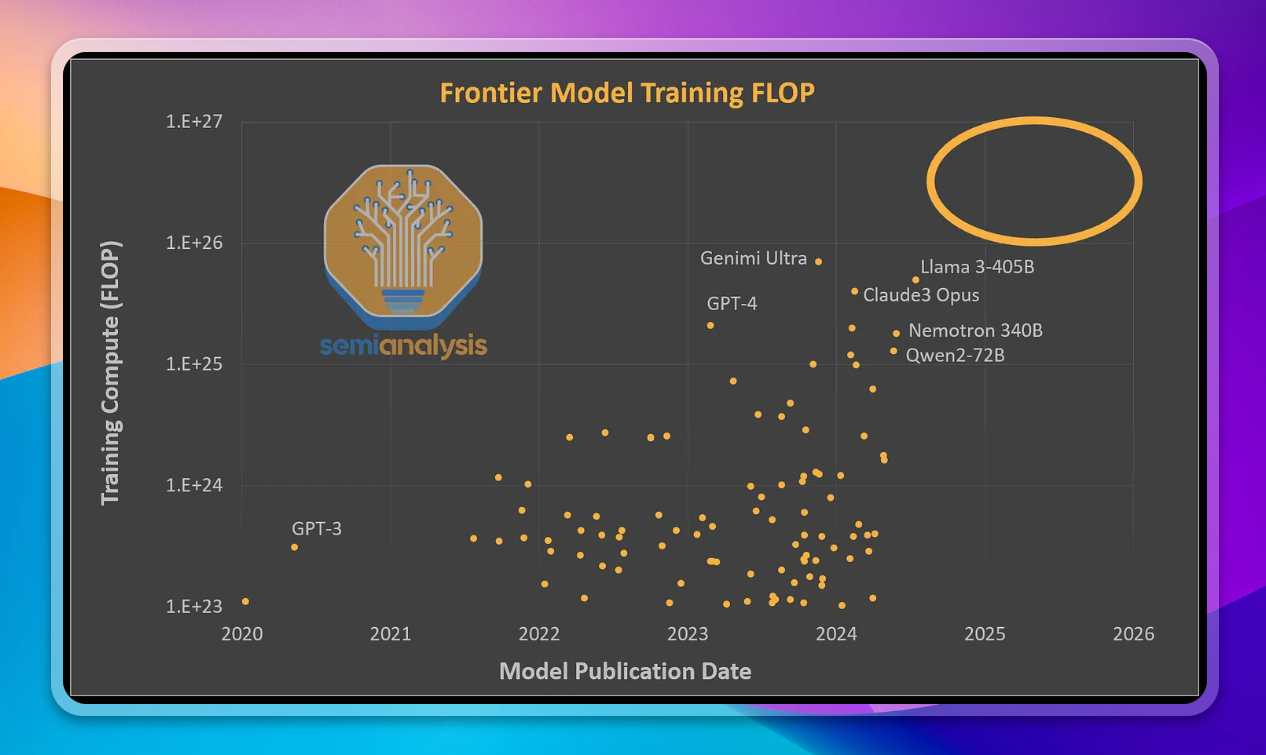
LLMs haven’t significantly improved since GPT4: is progress slowing? 🐢
Dramatically more powerful model training clusters are being built: 15 of them, with 31 times more power than trained GPT4
This means models much more powerful than GPT4 are coming 🐇
SemiAnalysis did a phenomenal deep dive into this topic - and it’s worth reading
GPT4 was trained several years ago, and model performance hasn’t significantly improved since then. They explain that this is primarily due to a stagnation of the compute allocated to model training - and that this is likely to change.
Despite some models - like Google Gemini Ultra and Nvidia Nemotron 340B - being trained with more compute than GPT4, they weren’t significantly better due to inferior architecture
GPT4 was trained on approximately 20,000 A100 GPUs, but new cluster sizes of 100,000 H100 GPUs will have approx 31x more compute capacity. This is a huge increase - these new clusters could train GPT4 in just 4 days, compared to the 90 days it took.
This will lead to new models with significant improvements in performance.
These new clusters are HUGE - and they’ll be incredibly expensive. Each one will cost over $4 billion, and require 150MW of power - enough for 100-200,000 US households.
Some people will argue this is a bad use of capital and energy, and that these could be better spent elsewhere. I disagree, and think AI progress will be enormous for productivity rates and economic growth. But will they be good investments for the companies developing them? That is less clear, given the incredible expense involved and the competitiveness of the space.
When compute is no longer the bottleneck to the development of more powerful models, what will be? Data quality and availability is likely to be one big hurdle, as will model architectures - and diminishing returns from additional compute.
So when should we expect these significantly better models? Unfortunately only the model developers know that.